As organizations go through digital transformation, the cyber challenges they face become more important. Their IT systems and applications become more critical and at the same time more open. The recent data breach suffered by British Airways illustrates the sophistication of the cyber adversaries and the difficulties faced by organization to prevent, detect, and respond to these challenges. One approach that is gaining ground is the application of AI technologies to cyber security and, at a London event in September 24, IBM described how IBM Watson is being integrated with other IBM security products to meet these challenges.
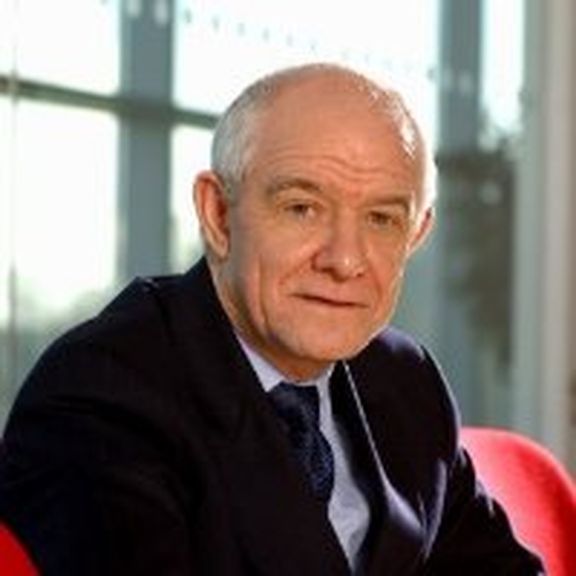
The current approaches to cyber defense include multiple layers of protection including firewalls and identity and access management as well as event monitoring (SIEM). While these remain necessary they have not significantly reduced the time to detect breaches. For example, the IBM-sponsored 2018 Cost of a Data Breach Study by Ponemon showed that the mean time for organizations to identify a breach was 197 days. This length of time has hardly improved over many years. The reasons for this long delay are many and include: the complexity of the IT infrastructure, the sophistication of the techniques used by cyber adversaries to hide their activities and the sheer volume data available.
So, what is AI and how can it help to mitigate this problem?
AI is a generic term that covers a range of technologies. In general, the term AI refers to systems that “simulate thought processes to assist in finding solutions to complex problems through augmentation and enhancement of human capabilities”. Kuppingercole has analyzed in detail what this really means in practice and this is summarized in the following slide from the EIC 2017 Opening Keynote by Martin Kuppinger.
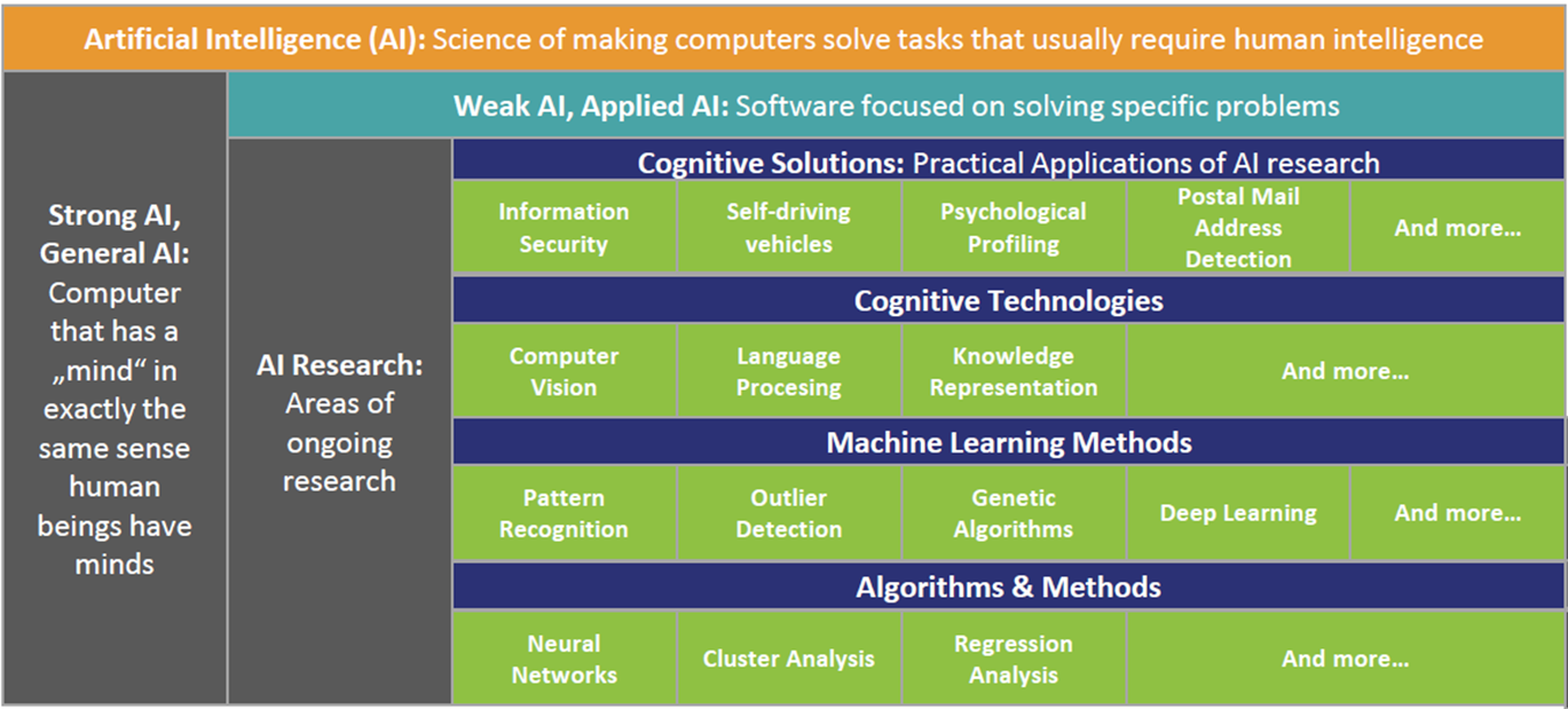
At the lower layer, improved algorithms enable the transformation of Big Data into “Smart Information”. See KuppingerCole Advisory Note: Big Data Security, Governance, Stewardship - 72565. This is augmented by Machine Learning where human reinforcement is used to tune the algorithms to identify those patterns that are of interest and to ignore those that are not. Cognitive technologies add an important element to this mix through their capability to include speech, vision and unstructured data into the analysis. Today, this represents the state of the art for the practical application of AI to cyber security.
The challenges of AI at the state of the art are threefold:
Cyber security products collect vast amounts of data – the cyber security analyst is literally drowning in data. The challenge is to find the so called IOCS (Indicators of Compromise), that show the existence of a real threat, amongst this enormous amount of data. The problem is to not just to find what is abnormal, but to filter out the many false positives that obscure the real threats.
There are several vendors that have incorporated Machine Learning (ML) systems into their products to tune the identification of important anomalies. This is useful to reduce false positives, but it is not enough. To be really useful to a security analyst, the abnormal pattern needs to be related to known or emerging threats. While there have been several attempts to standardize the way information on threats is described and shared most of this information is still held in unstructured form in documents, blogs and twitter feeds. It is essential to take account of these.
This is where IBM QRadar Advisor with Watson is different. A Machine Learning system is only as is only as good as its training – training is the key to its effectiveness. IBM say that it has been trained through the ingestion of over 10 billion pieces of structured data and 1.24 million unstructured documents to assist with the investigation of security incidents. This training involved IBM X-Force experts as well as IBM customers. Because of this training, it can now identify patterns that represents potential threats and provide links to the relevant sources that have been used to reach these conclusions. However, while this helps the security analyst to do their job more efficiently and more effectively, it does not yet replace the human.
Organizations now need to assume that cyber adversaries have access to their organizational systems and to constantly monitor for this activity in a way that will enable them to take action before damage is done. AI provides a great potential to help with this challenge and to evolve to help organizations to improve their cyber security posture through intelligent code analysis and configuration scanning as well as activity monitoring. For more information on the future of cyber security attend KuppingerCole’s Cybersecurity Leadership Summit 2018 Europe.
Mike Small is a senior analyst at KuppingerCole. Read more KuppingerCole blogs here.