Typical hybrid cloud IT integration strategies have fundamental design flaws that CIOs and CISOs need to address if they’re going to avert another attack on the scale of SolarWinds Orion beach, which was disclosed in December 2020.
The design flaws are evident in existing approaches to integrating public and private clouds with legacy systems. Inconsistent endpoint security and privileged access management has turned out to be highly penetrable and painfully lacking.
The first two articles in this series explain how getting hybrid cloud security right is hard and how the SolarWinds hack exposed hybrid clouds’ greatest weaknesses. This post lays out an approach to solve hybrid cloud security challenges today.
Finding security gaps with network maps
The best first step to improving hybrid cloud security is to gain an accurate, real-time view of every public, private, and community cloud and its integrations into legacy systems. The goal is to gain greater visibility and control across the entire network by continually capturing data on network activity down to the endpoint. Applying machine learning algorithms and cyber terrain analysis to the data uncovers security gaps hidden in data logs or points to openings where data is not captured at all.
Network mapping strategy must focus on quantifying how data moves within and between hybrid platforms. Hidden in the terabytes of data that hybrid clouds generate are indicators of potential vulnerabilities, and — in worst cases — anomalous activity indicating a breach attempt.
Comprehensive network maps that range down to the IP address level, combined with a network’s activity data, can identify potential security gaps. A data-centric approach based on real-time monitoring of a hybrid cloud network identifies the most vulnerable systems, network connections, and endpoints.
Real-time network monitoring also proves more effective than unifying the completely different monitoring approaches every public cloud platform has. Please don’t believe the hype from cloud platform providers that claim to support visibility across third-party cloud platforms and secure a hybrid cloud configuration. It’s best to take an impartial, independent strategy when it comes to network mapping a hybrid cloud configuration, ideally choosing a monitoring platform that delivers real-time data monitoring too.
Four Areas to Focus
Look for these four core areas of expertise when evaluating hybrid cloud mapping and security analysis platforms.
First, understand that, at a minimum, any cyber risk modeling platform needs to identify and isolate device endpoint vulnerabilities at the physical level of the work. It’s essential that a mapping platform supports this, because the telemetry data this generates is the foundation for creating an accurate network map.
Second, networking mapping platforms need to identify if each endpoint is up to date when it comes to patch management, where the endpoint is in the configuration structure of the hybrid cloud network, and what the potential vulnerabilities are, down to the level of the operating system and endpoint security patches.
Third, an effective network mapping platform can track each device down to the IP address, providing contextual intelligence and locational data.
Fourth, any network mapping platform needs to excel at visualization and provide insightful analysis at a graphical level to identify potential security anomalies and actual breach activity.
Useful in understanding this is the following example of how RedSeal’s cyber risk modeling software for hybrid cloud environments works. Cisco has standardized on this approach to identify security gaps in their hybrid cloud strategies and optimize hybrid cloud network performance.
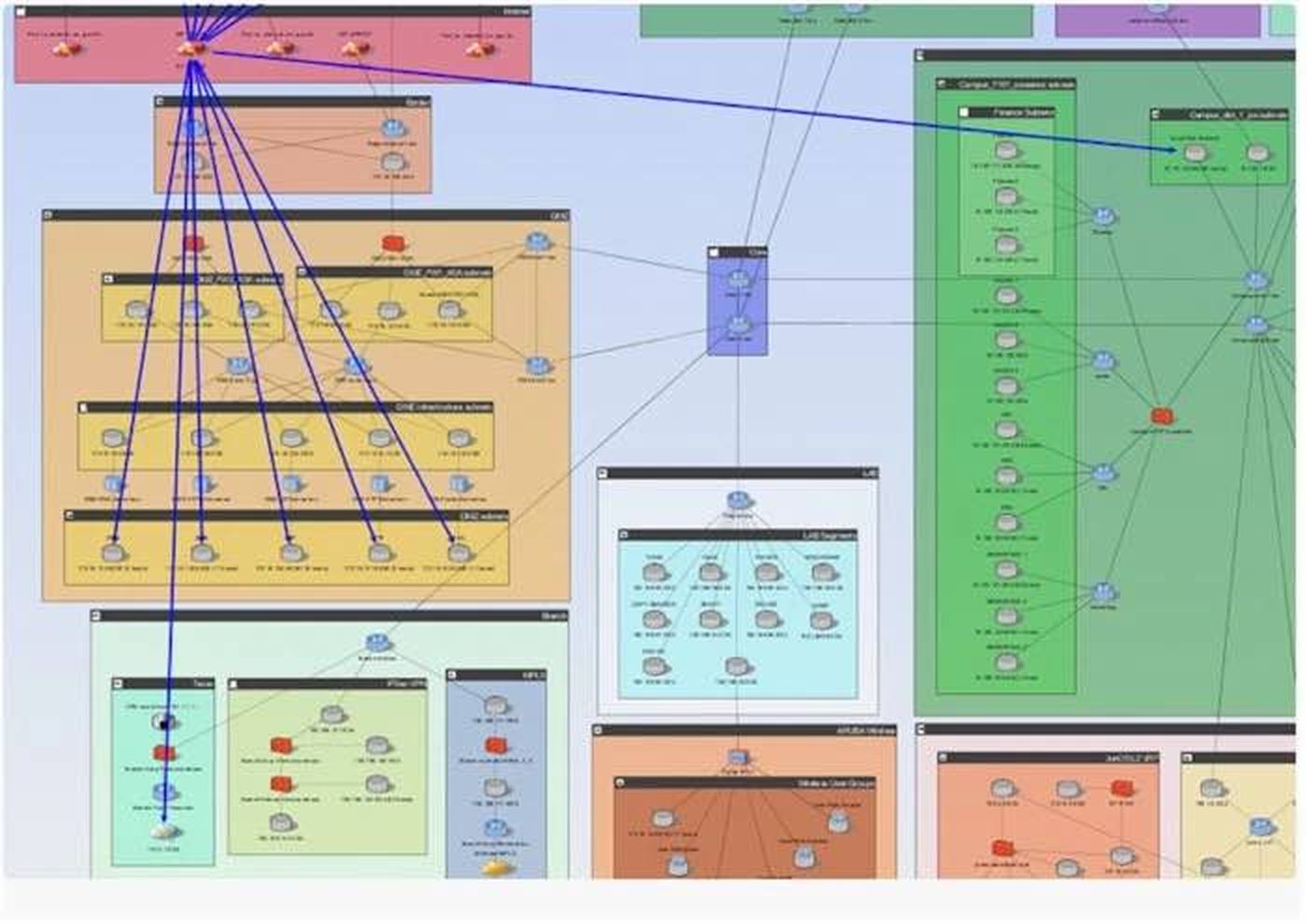
Machine learning identifies network vulnerabilities
Machine learning models are proving effective at identifying security gaps in hybrid cloud networks. That’s being accomplished by combining supervised and unsupervised algorithms to identify anomalies and create new predictive models based on results. The value of having real-time monitoring data obtained from network mapping starts to pay off when risk and threat correlation engines provide terrain mapping data and visualizations of a hybrid cloud network. Flaws, gaps, overlooked security configurations, and potential breach attempts are faster to find and remediate using machine learning analysis and visualization techniques.
Machine learning’s impact on hybrid cloud network mapping and vulnerability assessment has led some to create threat reference libraries. These compare configurations using threat correlation engines. By capitalizing on the insights gained from supervised machine learning models continually learning based on real-time data monitoring, threat correlation engines prove to be accurate in identifying breach attempts and anomalous activity. For organizations pursuing a hybrid cloud infrastructure strategy to support new businesses and services, that’s welcome news.
Paralleling the development of correlation engines are risk engines that capitalize on the data captured from real-time network monitoring. Risk engines use advanced predictive analytics to calculate the relative risk levels posed by unique combinations of hosts. By employing algorithms to cycle through multiple scenarios involving randomized hosts, these risk engines identify the most critical vulnerabilities. From there, risk scores define a prioritized list of vulnerabilities that need security teams’ immediate attention.
Cyber terrain analytics combines risk and threat correlation engines’ results, continually refining them using real-time network monitoring data. Over time, machine learning algorithms supporting the two engines fine-tune terrain analytics to quantify how resilient a hybrid cloud network is while also identifying vulnerabilities. The approach is proving effective in identifying threats in real time and taking action to thwart breach attempts in hybrid cloud configurations that would otherwise go undetected. Terrain analytics effectively model or simulate threat scenarios, providing invaluable data to organizations focused on hardening their hybrid cloud configurations.
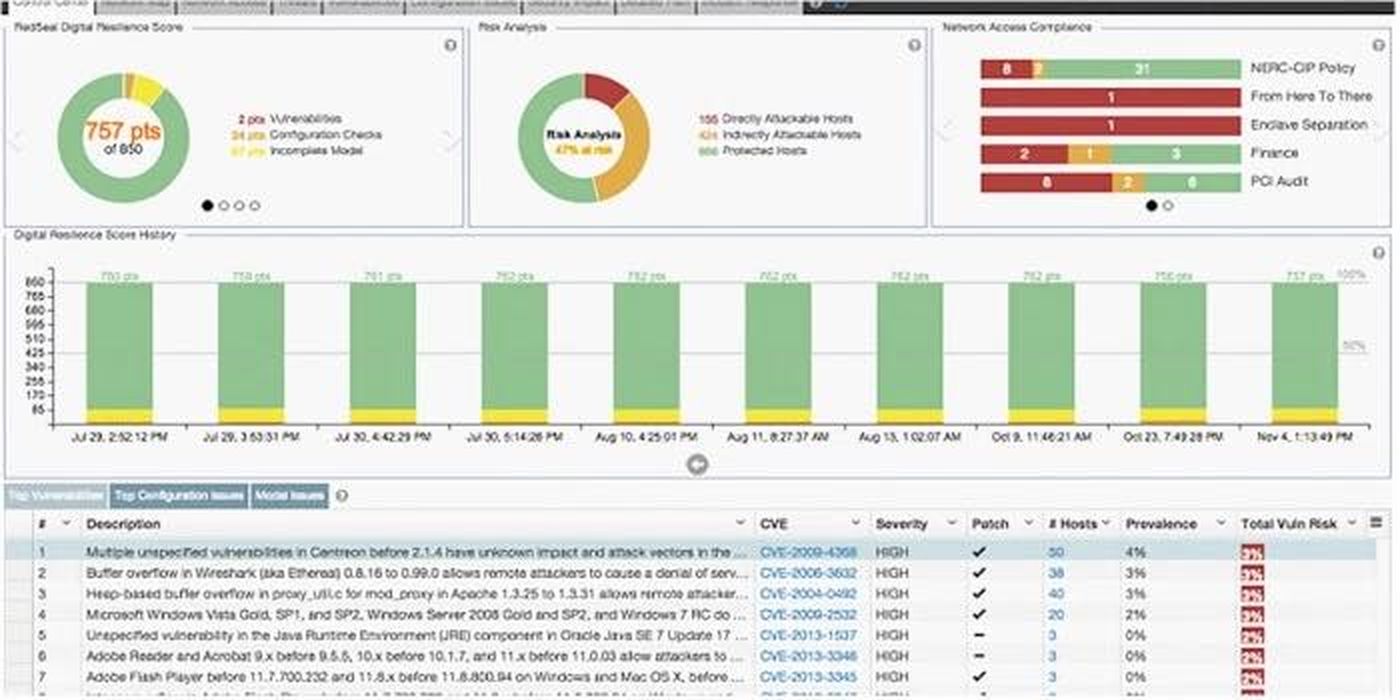
Answers lurk in the real-time data streams
Hybrid clouds’ greatest security weaknesses haven’t been discovered yet. That’s because they’re being managed for the most part with security techniques and tools that are decades old and were made for a time when business models were much simpler.
Today we need a more data-centric approach to security for hybrid cloud infrastructure, one that combines the best of what data governance can provide with the latest machine learning technologies for identifying and acting on vulnerabilities.
The answers to how to improve hybrid cloud security are hidden in the real-time data streams these platforms produce as they operate and interact with both valid internal users and bad actors attempting to breach the system. Creating a contextual intelligence, along with a real-time view of all hybrid cloud activity, is where it needs to start.
Author Louis Columbus is principal at Dassault Systemes, an adjunct professor at Webster University, and a contributor to such sites as VentureBeat and Entrepreneur Media. Blog courtesy of RedSeal. Read more RedSeal guest blogs here.